Source: Judea Pearl, "Theoretical Impediments to Machine Learning with Seven Sparks from the Causal Revolution," avail. online at arXiv (Jan. 2018)
Context
Many are cramming through the LLM gates, perhaps ignorant of the casual machine learning revolution...
Two quotes to situate the moment:
- Rigid Babylonians vs. Wild Greeks
"Babylonians [sic] astronomers were masters of black-box prediction, far surpassing their Greek rivals in accuracy and consistency. Yet Science favored the creative-speculative strategy of the Greek astronomers which was wild with metaphorical imagery: circular tubes full of fire, small holes through which celestial fire was visible as stars, and hemispherical earth riding on turtle backs.
Yet it was this wild modeling strategy, not Babylonian rigidity, that jolted Eratosthenes (276-194 BC) to perform one of the most creative experiments in the ancient world and measure the radius of the earth. This would never have occurred to a Babylonian curve-fitter." (Pearl at 7) - Model-Blindness vs. Model<>Data Symbiosis
"Our general conclusion is that human-level AI cannot emerge solely from model-blind learning machines; it requires the symbiotic collaboration of data and models... Data alone are hardly a science, regardless of how big they get and how skillfully they are manipulated. (Id. at 7)
The Map.
The map is never the territory, but that does not mean one should ignore the map.
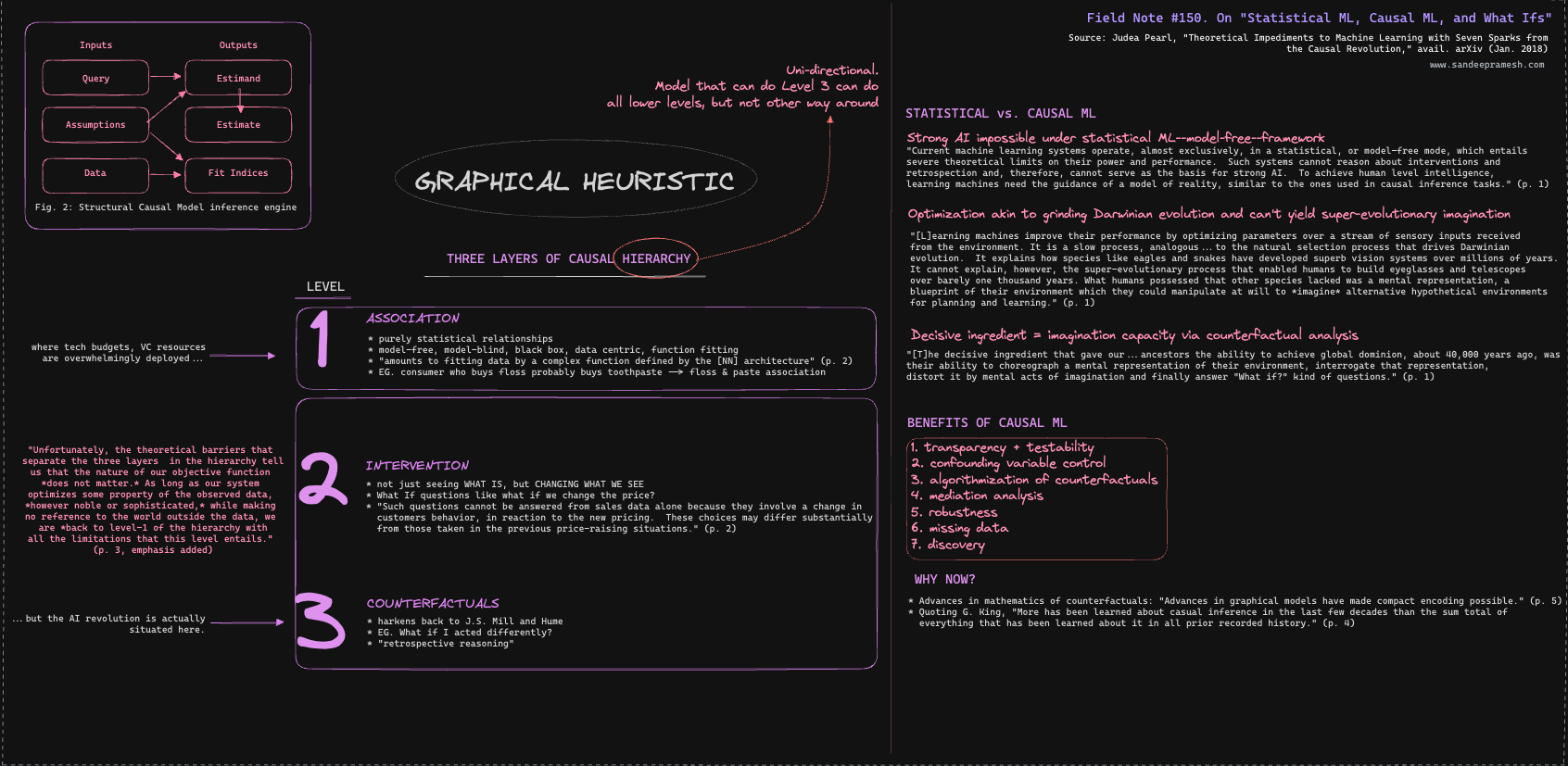